Which Data Science Algorithms Should You Be Leveraging Right Now?
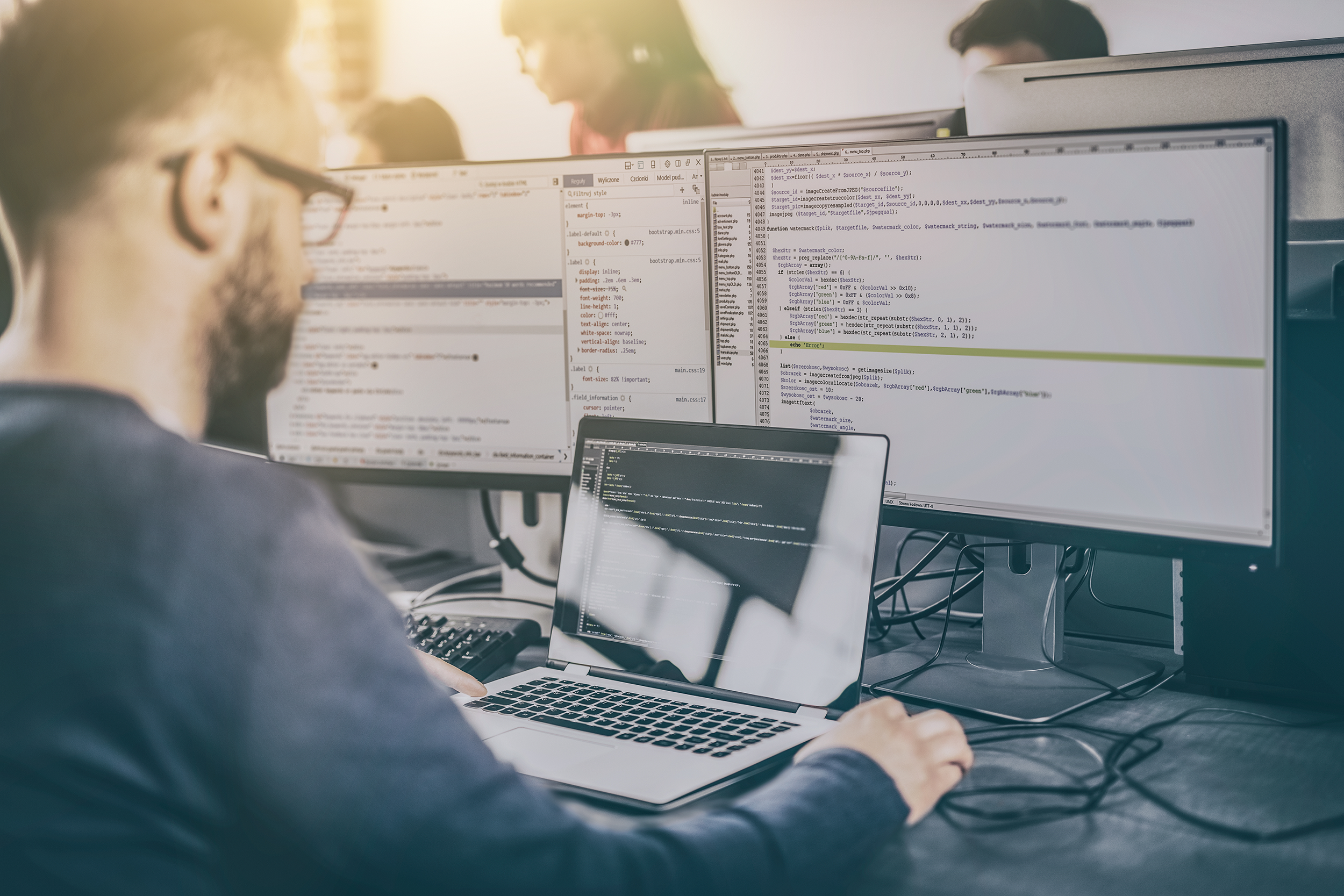
With Wesfarmers having a strategic priority of developing a market leading data and digital ecosystem, the ability to combine the smart selection and application of data science algorithms with our rich and diverse data asset is key to our future success, and so a focus of the Data Scientists in the Wesfarmers Advanced Analytics Centre (AAC).
In essence, our role as data scientists is to help businesses answer questions and solve problems through insights obtained via their data.
Now, with data collection and reporting methods increasing rapidly in both volume and sophistication, businesses want those questions answered more efficiently and accurately, and expect problems to be solved in a scalable and automated fashion.
And the best way we can do that as data scientists is by using algorithms.
It’s true that the amount of algorithms available is exploding every day, but ultimately, the one that’s most relevant to you and your objectives isn’t necessarily the latest one out there, or any specifically designated one – it’s just a matter of finding the one that solves your problem in the quickest and most accurate way, with the least complexity.
That’s not to say that certain types of algorithms aren’t becoming more widely used than others. For instance, recommender-based systems for prediction, as well as RNNs, are increasingly important and popular amongst the DS community. However, more often than not, it is possible to create a scenario where you can indeed make a particular algorithm you want to use fit with the business problem you are trying to address. Keeping this in mind, there is a case to be made that there are potentially many algorithms vying for the "most relevant" to any particular problem.
Above all, what’s most important is that you are using algorithms you understand well and are explainable: being comfortable with the intricacies of how an algorithm behaves – the bounds of its performance, what is normal and not, its pros and cons – can be the deciding factor in how well an algorithm works when applying it to a specific situation.
Topic modelling for customer purchasing data at Wesfarmers
Thanks to the use of data algorithms at Wesfarmers, we now understand how we can better serve customer needs through investigating what they are interested in. We’re doing this by delivering a 360-degree view of how a customer behaves when making different decisions, grouping together different customer behaviours algorithmically. Our method of tackling this is by using ‘topic modelling’.
Topic modelling is a recommender-based system that finds connections between similar purchasing customers to help assign affinities to these overarching topics of purchasing behaviour. The aim is to create different 'topics' of user preferences, based on what they have bought across the Wesfarmers divisions.
This topic modelling is really powerful as it enables us to not only group together customer purchasing behaviour, but also to identify when customers belong to these groups, who we wouldn’t have identified previously.
What’s more, due to the model’s like-for-like ability, we can discern when a customer may have a high affinity for a category of purchasing, even though they have never bought anything specifically from a certain category. This is key to cross and up-selling and therefore has potential to add huge value through increasing the relevance of customer communications and experiences across the Wesfarmers retail brands.
The data science innovation I’d love to see over the next few years
Looking ahead, my vision is for more data science algorithms to take into account a more holistic view of the ecosystem they are operating in. We often end up making decisions through the use of data science, with a level of data that represents just a fraction of the total information potentially available.
For example, when we are trying to figure out why a customer acted in a certain way, it is important to realise we could be missing some data that would explain it – our models might not have access to crucial pieces of information that would have helped us to make that prediction. Thinking that our model(s) are the one and only solution to a vast and challenging problem set is setting ourselves up to fail.
Obviously humans behave unpredictably sometimes, and that's normal! But I’d love to see data science algorithms able to capture this in some way and somehow represent the data they may be missing, so we can understand this interplay better. I believe this will be key to improving the data science industry as a whole.
This is one of the many challenges that the data science community across the Wesfarmers Group are seeking to navigate and solve – and so make a positive difference to our customers lives through helping them discover new products and experiences and better anticipate their needs.
Are you looking to explore a career in data science? If so I’d encourage you to head over to our careers site to find out more about the opportunities available through our Advanced Analytics Centre.

